How Driscoll’s is Embracing AI to Build an Even Better Berry
Driscoll’s has been a leader in the fresh berry category for more than a century. The company is a household name in a competitive category by continuously looking for ways to improve flavor, freshness and consistency to delight consumers around the world while enabling independent farmers to thrive.
Driscoll’s is an early adopter of new ideas and, unlike many brands that grow generic varieties, Driscoll’s provides its own proprietary baby plants to its growers, and then buys the fruit back to be inspected, cooled, packaged, marketed and shipped to grocery stores.
The Problem
Driscoll’s invests heavily in technologies like AI and machine learning (ML). But implementing AI in an agribusiness as complex as Driscoll’s is not a one-time task or a simple software-as-a-service (SaaS) project. AI models need constant retraining and curation and are hungry for data. Implementing domain-specific AI, such as agriculture, requires close collaboration between experts and the engineers and data scientists selecting the models and designing the feedback systems.
To rapidly accelerate its AI-driven approach, Driscoll’s activated a partner with deep AI expertise and tech and an exclusive focus on agriculture. Enter Mineral.
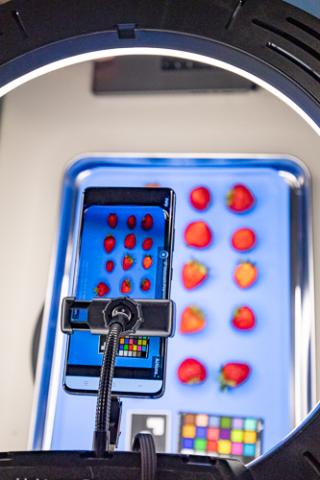
The Solutions
Driscoll’s first engaged the Mineral team to explore ways to improve data collection in its breeding operations. Crop breeding involves the careful collection of multiple data points about each plant variety throughout the growing season. To that end, Driscoll’s breeding team is pioneering ways to use robotics and AI to measure plants’ traits — such as the length of strawberry stems or the size of individual raspberries — on thousands of plants in trial plots. The ability to collect data at scale continuously through the season — in particular, data that’s tedious to measure manually — frees up breeders to focus on other tasks.
Insights gained from this data inspired Driscoll’s to work with Mineral engineers on another challenge: predicting the yield of each plant. Accurate yield forecasting is incredibly important in the berry industry, as it drives critical decisions such as pricing, volume allocation to partners, packaging needs and labor availability. But growing is a natural system influenced by the soil, weather, farmer decisions, the time of the season, pest and disease pressure, and hundreds of other variables, making it challenging and time-consuming to produce accurate forecasts.
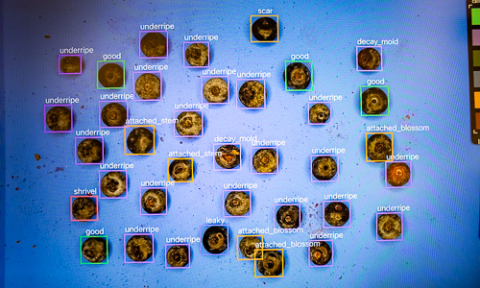
Integrating hundreds of different data types, including time-series data, is well suited to the power of AI. By working hand in hand with Mineral’s engineers and data scientists, Driscoll’s is able to significantly improve its forecast accuracy, increase the frequency of forecasting, reduce forecast volatility and extend its forecasting horizon. Most exciting is that the AI models are creating a deeper understanding about the drivers of berry yield.
A key learning from this initiative is the synergy of human experts and ML algorithms. Mineral’s AI model was able to crunch billions of data points about weather and discover subtle patterns in historical trends, but was easily flummoxed by an unusual pest outbreak. Driscoll’s forecasters, however, are experts in the nuances of berry cultivation and can call a grower to get up-to-date information. The combination of human intuition and raw AI power delivered a more accurate forecast than either alone. Each system learned from the other.
The Results: The Never-Ending Pursuit of Delight
Another spinoff from the breeding work was the realization that AI could accurately and consistently measure fruit quality. Driscoll’s expert quality raters inspect berries when they arrive from the field and before they’re cooled for shipping. These inspectors need to accurately rate millions of berries annually, correctly identifying dozens of defects. This quality control not only affects grower payments, but also provides critical insights into the performance of varieties and the berries’ shelf life.
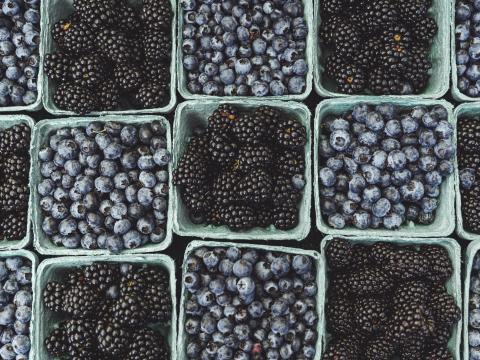
Working closely with Mineral’s powerful perception tools and AI team, Driscoll’s built a system that performed similarly to human experts, unlocking the opportunity to extend the technology across its complex ecosystem. Not only did the AI algorithms quickly learn the subtleties of berry defects, but the success also inspired new ideas for how AI could transform other parts of Driscoll’s business.
The breeding project tasked robots to gather data from millions of plants, freeing up workers to focus on higher-level tasks. The yield forecasting project improved accuracy, frequency and a longer prediction horizon. And the quality control project enabled the expertise of inspectors to be replicated elsewhere in the supply chain. But perhaps the most important outcome of this trio of projects lies in the way that the discoveries build upon one another. In this way, AI is not just a tool for solving a known problem, but also an engine with the potential to generate entirely new ways of doing business.
Wondering what new intelligence Mineral might help you unlock? Read more about Mineral’s philosophy on partnership, or reach out directly.